We study the linear convergence of the primal-dual hybrid gradient method. After a review of current analyses, we show that they do not explain properly the behavior of the algorithm, even on the most simple problems. We thus introduce the quadratic error bound of the smoothed gap, a new regularity assumption that holds for a wide class of optimization problems. Equipped with this tool, we manage to prove tighter convergence rates. Then, we show that averaging and restarting the primal-dual hybrid gradient allows us to leverage better the regularity constant. Numerical experiments on linear and quadratic programs, ridge regression and image denoising illustrate the findings of the paper.
Revised:
Accepted:
Published online:
Olivier Fercoq 1
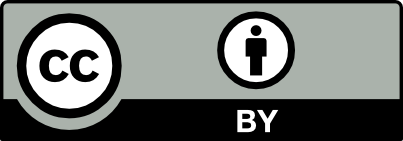
@article{OJMO_2023__4__A6_0, author = {Olivier Fercoq}, title = {Quadratic error bound of the smoothed gap and the restarted averaged primal-dual hybrid gradient}, journal = {Open Journal of Mathematical Optimization}, eid = {6}, pages = {1--34}, publisher = {Universit\'e de Montpellier}, volume = {4}, year = {2023}, doi = {10.5802/ojmo.26}, language = {en}, url = {https://ojmo.centre-mersenne.org/articles/10.5802/ojmo.26/} }
TY - JOUR AU - Olivier Fercoq TI - Quadratic error bound of the smoothed gap and the restarted averaged primal-dual hybrid gradient JO - Open Journal of Mathematical Optimization PY - 2023 SP - 1 EP - 34 VL - 4 PB - Université de Montpellier UR - https://ojmo.centre-mersenne.org/articles/10.5802/ojmo.26/ DO - 10.5802/ojmo.26 LA - en ID - OJMO_2023__4__A6_0 ER -
%0 Journal Article %A Olivier Fercoq %T Quadratic error bound of the smoothed gap and the restarted averaged primal-dual hybrid gradient %J Open Journal of Mathematical Optimization %D 2023 %P 1-34 %V 4 %I Université de Montpellier %U https://ojmo.centre-mersenne.org/articles/10.5802/ojmo.26/ %R 10.5802/ojmo.26 %G en %F OJMO_2023__4__A6_0
Olivier Fercoq. Quadratic error bound of the smoothed gap and the restarted averaged primal-dual hybrid gradient. Open Journal of Mathematical Optimization, Volume 4 (2023), article no. 6, 34 p. doi : 10.5802/ojmo.26. https://ojmo.centre-mersenne.org/articles/10.5802/ojmo.26/
[1] On the convergence of stochastic primal-dual hybrid gradient (2019) (https://arxiv.org/abs/1911.00799)
[2] Linear convergence of primal–dual gradient methods and their performance in distributed optimization, Automatica, Volume 117 (2020), 109003 | MR | Zbl
[3] Faster First-Order Primal-Dual Methods for Linear Programming using Restarts and Sharpness (2021) (https://arxiv.org/abs/2105.12715)
[4] et al. Convex analysis and monotone operator theory in Hilbert spaces, 408, Springer, 2011 | DOI
[5] From error bounds to the complexity of first-order descent methods for convex functions, Math. Program., Volume 165 (2017) no. 2, pp. 471-507 | DOI | MR | Zbl
[6] A first-order primal-dual algorithm for convex problems with applications to imaging, J. Math. Imaging Vis., Volume 40 (2011) no. 1, pp. 120-145 | DOI | MR | Zbl
[7] LIBSVM: a library for support vector machines, ACM Trans. Intell. Syst. Technol., Volume 2 (2011) no. 3, pp. 1-27 | DOI
[8] A primal–dual splitting method for convex optimization involving Lipschitzian, proximable and linear composite terms, J. Optim. Theory Appl., Volume 158 (2013) no. 2, pp. 460-479 | DOI | MR | Zbl
[9] Proximal splitting algorithms: A tour of recent advances, with new twists (2019) (https://arxiv.org/abs/1912.00137)
[10] Convergence rate analysis of several splitting schemes, Splitting methods in communication, imaging, science, and engineering (Scientific Computation), Springer, 2016, pp. 115-163 | DOI | Zbl
[11] Implicit functions and solution mappings. A view from variational analysis, Springer Monographs in Mathematics, Springer, 2009 | DOI
[12] Error bounds, quadratic growth, and linear convergence of proximal methods, Math. Oper. Res., Volume 43 (2018) no. 3, pp. 919-948 | DOI | MR | Zbl
[13] Linear convergence of the primal-dual gradient method for convex-concave saddle point problems without strong convexity, The 22nd International Conference on Artificial Intelligence and Statistics, PMLR (2019), pp. 196-205
[14] A coordinate-descent primal-dual algorithm with large step size and possibly nonseparable functions, SIAM J. Optim., Volume 29 (2019) no. 1, pp. 100-134 | DOI | MR | Zbl
[15] Adaptive restart of accelerated gradient methods under local quadratic growth condition, IMA J. Numer. Anal., Volume 39 (2019) no. 4, pp. 2069-2095 | DOI | MR | Zbl
[16] Restarting the accelerated coordinate descent method with a rough strong convexity estimate, Comput. Optim. Appl., Volume 75 (2020) no. 1, pp. 63-91 | DOI | MR | Zbl
[17] A dual algorithm for the solution of nonlinear variational problems via finite element approximation, Comput. Math. Appl., Volume 2 (1976) no. 1, pp. 17-40 | DOI | Zbl
[18] On Approximate Solutions of Systems of Linear Inequalities, Journal of Research of the National Bureau of Standards, Volume 49 (1952) no. 4, p. 263 | DOI | MR
[19] Unified linear convergence of first-order primal-dual algorithms for saddle point problems, Optim. Lett., Volume 16 (2022) no. 6, pp. 1675-1700 | DOI | MR | Zbl
[20] Optimal and practical algorithms for smooth and strongly convex decentralized optimization, Adv. Neural Inf. Process. Syst., Volume 33 (2020)
[21] A new randomized block-coordinate primal-dual proximal algorithm for distributed optimization, IEEE Trans. Autom. Control, Volume 64 (2019) no. 10, pp. 4050-4065 | DOI | MR | Zbl
[22] Convergence rates with inexact non-expansive operators, Math. Program., Volume 159 (2016) no. 1-2, pp. 403-434 | DOI | MR | Zbl
[23] First-Order Methods for Convex Constrained Optimization under Error Bound Conditions with Unknown Growth Parameters (2020) (https://arxiv.org/abs/2010.15267)
[24] An adaptive proximal point algorithm framework and application to large-scale optimization (2020) (https://arxiv.org/abs/2008.08784)
[25] Smooth minimization of non-smooth functions, Math. Program., Volume 103 (2005) no. 1, pp. 127-152 | DOI | MR | Zbl
[26] et al. Lectures on convex optimization, 137, Springer, 2018 | DOI
[27] Lower complexity bounds of first-order methods for convex-concave bilinear saddle-point problems, Math. Program., Volume 185 (2021) no. 1-2, pp. 1-35 | DOI | MR | Zbl
[28] Variational analysis, 317, Springer, 2009
[29] Dualize, split, randomize: Toward fast nonsmooth optimization algorithms, J. Optim. Theory Appl., Volume 195 (2022) no. 1, pp. 102-130 | DOI | MR | Zbl
[30] A smooth primal-dual optimization framework for nonsmooth composite convex minimization, SIAM J. Optim., Volume 28 (2018) no. 1, pp. 96-134 | DOI | MR | Zbl
[31] A splitting algorithm for dual monotone inclusions involving cocoercive operators, Adv. Comput. Math., Volume 38 (2013) no. 3, pp. 667-681 | MR | Zbl
[32] Linear Convergence of Randomized Primal-Dual Coordinate Method for Large-scale Linear Constrained Convex Programming, International Conference on Machine Learning, PMLR (2020), pp. 11619-11628
Cited by Sources: