We study the convergence rate of Bregman gradient methods for convex optimization in the space of measures on a -dimensional manifold. Under basic regularity assumptions, we show that the suboptimality gap at iteration is in for multiplicative updates, while it is in for additive updates for some determined by the structure of the objective function. Our flexible proof strategy, based on approximation arguments, allows us to painlessly cover all Bregman Proximal Gradient Methods (PGM) and their acceleration (APGM) under various geometries such as the hyperbolic entropy and divergences. We also prove the tightness of our analysis with matching lower bounds and confirm the theoretical results with numerical experiments on low dimensional problems. Note that all these optimization methods must additionally pay the computational cost of discretization, which can be exponential in .
Accepted:
Published online:
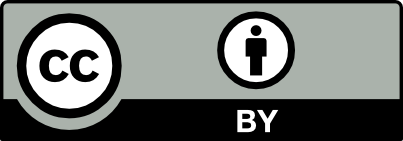
@article{OJMO_2022__3__A8_0, author = {L\'ena{\"\i}c Chizat}, title = {Convergence {Rates} of {Gradient} {Methods} for {Convex} {Optimization} in the {Space} of {Measures}}, journal = {Open Journal of Mathematical Optimization}, eid = {8}, pages = {1--19}, publisher = {Universit\'e de Montpellier}, volume = {3}, year = {2022}, doi = {10.5802/ojmo.20}, language = {en}, url = {https://ojmo.centre-mersenne.org/articles/10.5802/ojmo.20/} }
TY - JOUR AU - Lénaïc Chizat TI - Convergence Rates of Gradient Methods for Convex Optimization in the Space of Measures JO - Open Journal of Mathematical Optimization PY - 2022 SP - 1 EP - 19 VL - 3 PB - Université de Montpellier UR - https://ojmo.centre-mersenne.org/articles/10.5802/ojmo.20/ DO - 10.5802/ojmo.20 LA - en ID - OJMO_2022__3__A8_0 ER -
%0 Journal Article %A Lénaïc Chizat %T Convergence Rates of Gradient Methods for Convex Optimization in the Space of Measures %J Open Journal of Mathematical Optimization %D 2022 %P 1-19 %V 3 %I Université de Montpellier %U https://ojmo.centre-mersenne.org/articles/10.5802/ojmo.20/ %R 10.5802/ojmo.20 %G en %F OJMO_2022__3__A8_0
Lénaïc Chizat. Convergence Rates of Gradient Methods for Convex Optimization in the Space of Measures. Open Journal of Mathematical Optimization, Volume 3 (2022), article no. 8, 19 p. doi : 10.5802/ojmo.20. https://ojmo.centre-mersenne.org/articles/10.5802/ojmo.20/
[1] , Conference on Learning Theory (2020), pp. 163-182
[2] Interior gradient and proximal methods for convex and conic optimization, SIAM J. Optim., Volume 16 (2006) no. 3, pp. 697-725 | DOI | MR | Zbl
[3] On the Implicit Bias of Initialization Shape: Beyond Infinitesimal Mirror Descent (2021) (https://arxiv.org/abs/2102.09769)
[4] Breaking the curse of dimensionality with convex neural networks, J. Mach. Learn. Theory, Volume 18 (2017) no. 1, pp. 629-681
[5] Learning Theory from First Principles Draft, 2021
[6] Essential smoothness, essential strict convexity, and Legendre functions in Banach spaces, Commun. Contemp. Math., Volume 3 (2001) no. 04, pp. 615-647 | DOI | MR | Zbl
[7] Bregman monotone optimization algorithms, SIAM J. Control Optim., Volume 42 (2003) no. 2, pp. 596-636 | DOI | MR | Zbl
[8] A fast iterative shrinkage-thresholding algorithm for linear inverse problems, SIAM J. Imaging Sci., Volume 2 (2009) no. 1, pp. 183-202 | DOI | MR | Zbl
[9] , Advances in Neural Information Processing Systems, Volume 18 (2006)
[10] The alternating descent conditional gradient method for sparse inverse problems, SIAM J. Optim., Volume 27 (2017) no. 2, pp. 616-639 | DOI | MR | Zbl
[11] Linear convergence of iterative soft-thresholding, J. Fourier Anal. Appl., Volume 14 (2008) no. 5-6, pp. 813-837 | DOI | MR | Zbl
[12] Inverse problems in spaces of measures, ESAIM, Control Optim. Calc. Var., Volume 19 (2013) no. 1, pp. 190-218 | DOI | Numdam | MR | Zbl
[13] Convex Optimization: Algorithms and Complexity, Found. Trends Mach. Learn., Volume 8 (2015) no. 3-4, pp. 231-357 | DOI | Zbl
[14] Towards a mathematical theory of super-resolution, Commun. Pure Appl. Math., Volume 67 (2014) no. 6, pp. 906-956 | DOI | MR | Zbl
[15] "FISTA" in Banach spaces with adaptive discretisations (2021) (https://arxiv.org/abs/2101.09175)
[16] Sparse optimization on measures with over-parameterized gradient descent, Math. Program. (2021), pp. 1-46
[17] , Conference on Learning Theory (2020), pp. 1305-1338
[18] Fast projection onto the simplex and the ball, Math. Program., Volume 158 (2016) no. 1, pp. 575-585 | DOI | MR | Zbl
[19] Acceleration methods (2021) (https://arxiv.org/abs/2101.09545)
[20] Exact reconstruction using Beurling minimal extrapolation, J. Math. Anal. Appl., Volume 395 (2012) no. 1, pp. 336-354 | DOI | MR | Zbl
[21] The sliding Frank–Wolfe algorithm and its application to super-resolution microscopy, Inverse Probl., Volume 36 (2019) no. 1, 014001, 42 pages | DOI | MR | Zbl
[22] Stochastic approximation in Hilbert spaces, Ph. D. Thesis, PSL Research University (2017)
[23] , Advances in Neural Information Processing Systems, Volume 33 (2020), pp. 20215-20226
[24] Thresholding gradient methods in Hilbert spaces: support identification and linear convergence, ESAIM, Control Optim. Calc. Var., Volume 26 (2020), 28, 20 pages | DOI | MR | Zbl
[25] , Algorithmic Learning Theory (2020), pp. 386-407
[26] Riemannian geometry as determined by the volumes of small geodesic balls, Acta Math., Volume 142 (1979) no. 1, pp. 157-198 | DOI | MR | Zbl
[27] Mean-value theorems for Riemannian manifolds, Proc. R. Soc. Edinb., Sect. A, Math., Volume 92 (1982) no. 3-4, pp. 343-364 | DOI | MR | Zbl
[28] Solving large-scale optimization problems with a convergence rate independent of grid size, SIAM J. Numer. Anal., Volume 57 (2019) no. 3, pp. 1100-1123 | DOI | MR | Zbl
[29] The Moreau envelope function and proximal mapping in the sense of the Bregman distance, Nonlinear Anal., Theory Methods Appl., Volume 75 (2012) no. 3, pp. 1385-1399 | MR | Zbl
[30] Exponentiated gradient versus gradient descent for linear predictors, Inf. Comput., Volume 132 (1997) no. 1, pp. 1-63 | DOI | MR | Zbl
[31] Global optimization with polynomials and the problem of moments, SIAM J. Optim., Volume 11 (2001) no. 3, pp. 796-817 | DOI | MR | Zbl
[32] , Proceedings of the 27th International Conference on Neural Information Processing Systems-Volume 2 (2014), pp. 1970-1978
[33] A mean field view of the landscape of two-layer neural networks, Proc. Natl. Acad. Sci. USA, Volume 115 (2018) no. 33, p. E7665-E7671 | MR | Zbl
[34] Problem complexity and method efficiency in optimization, Wiley-Interscience series in discrete mathematics, John Wiley & Sons, 1983
[35] On an approach to the construction of optimal methods of minimization of smooth convex functions, Ekonom. i. Mat. Metody, Volume 24 (1988) no. 3, pp. 509-517
[36] Introductory lectures on convex optimization: A basic course, 87, Springer, 2003
[37] Particle Dual Averaging: Optimization of Mean Field Neural Networks with Global Convergence Rate Analysis (2020) (https://arxiv.org/abs/2012.15477)
[38] The geometry of off-the-grid compressed sensing (2018) (https://arxiv.org/abs/1802.08464)
[39] Integrals which are convex functionals. II, Pac. J. Math., Volume 39 (1971) no. 2, pp. 439-469 | DOI | MR | Zbl
[40] Approximation accuracy, gradient methods, and error bound for structured convex optimization, Math. Program., Volume 125 (2010) no. 2, pp. 263-295 | DOI | MR | Zbl
[41] , Advances in Neural Information Processing Systems, Volume 32 (2019)
[42] Can shallow neural networks beat the curse of dimensionality? A mean field training perspective, IEEE Trans. Artif. Intell., Volume 1 (2020) no. 2, pp. 121-129 | DOI
[43] On early stopping in gradient descent learning, Computing, Volume 26 (2007) no. 2, pp. 289-315 | MR | Zbl
[44] The strong convexity of Von Neumann’s entropy, 2013 (Unpublished note)
Cited by Sources: