In this work, we propose an adaptive variation on the classical Heavy-ball method for convex quadratic minimization. The adaptivity crucially relies on so-called “Polyak step-sizes”, which consists of using the knowledge of the optimal value of the optimization problem at hand instead of problem parameters such as a few eigenvalues of the Hessian of the problem. This method happens to also be equivalent to a variation of the classical conjugate gradient method, and thereby inherits many of its attractive features, including its finite-time convergence, instance optimality, and its worst-case convergence rates.
The classical gradient method with Polyak step-sizes is known to behave very well in situations in which it can be used, and the question of whether incorporating momentum in this method is possible and can improve the method itself appeared to be open. We provide a definitive answer to this question for minimizing convex quadratic functions, an arguably necessary first step for developing such methods in more general setups.
Revised:
Accepted:
Published online:
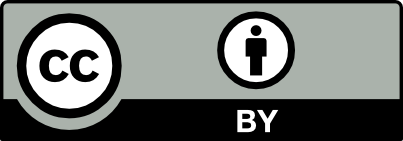
@article{OJMO_2024__5__A7_0, author = {Baptiste Goujaud and Adrien Taylor and Aymeric Dieuleveut}, title = {Short {Paper} - {Quadratic} minimization: from conjugate gradient to an adaptive {Polyak{\textquoteright}s} momentum method with {Polyak} step-sizes}, journal = {Open Journal of Mathematical Optimization}, eid = {9}, pages = {1--10}, publisher = {Universit\'e de Montpellier}, volume = {5}, year = {2024}, doi = {10.5802/ojmo.36}, language = {en}, url = {https://ojmo.centre-mersenne.org/articles/10.5802/ojmo.36/} }
TY - JOUR AU - Baptiste Goujaud AU - Adrien Taylor AU - Aymeric Dieuleveut TI - Short Paper - Quadratic minimization: from conjugate gradient to an adaptive Polyak’s momentum method with Polyak step-sizes JO - Open Journal of Mathematical Optimization PY - 2024 SP - 1 EP - 10 VL - 5 PB - Université de Montpellier UR - https://ojmo.centre-mersenne.org/articles/10.5802/ojmo.36/ DO - 10.5802/ojmo.36 LA - en ID - OJMO_2024__5__A7_0 ER -
%0 Journal Article %A Baptiste Goujaud %A Adrien Taylor %A Aymeric Dieuleveut %T Short Paper - Quadratic minimization: from conjugate gradient to an adaptive Polyak’s momentum method with Polyak step-sizes %J Open Journal of Mathematical Optimization %D 2024 %P 1-10 %V 5 %I Université de Montpellier %U https://ojmo.centre-mersenne.org/articles/10.5802/ojmo.36/ %R 10.5802/ojmo.36 %G en %F OJMO_2024__5__A7_0
Baptiste Goujaud; Adrien Taylor; Aymeric Dieuleveut. Short Paper - Quadratic minimization: from conjugate gradient to an adaptive Polyak’s momentum method with Polyak step-sizes. Open Journal of Mathematical Optimization, Volume 5 (2024), article no. 9, 10 p. doi : 10.5802/ojmo.36. https://ojmo.centre-mersenne.org/articles/10.5802/ojmo.36/
[1] Acceleration by stepsize hedging I: Multi-step descent and the silver stepsize schedule (2023) | arXiv
[2] Complexity guarantees for Polyak steps with momentum, Conference on Learning Theory, PMLR (2020), pp. 452-478
[3] Two-point step size gradient methods, IMA J. Numer. Anal., Volume 8 (1988) no. 1, pp. 141-148 | DOI | Zbl
[4] Accelerated gossip in networks of given dimension using Jacobi polynomial iterations, SIAM J. Math. Data Sci., Volume 2 (2020) no. 1, pp. 24-47 | DOI | Zbl
[5] Numerical optimization: theoretical and practical aspects, Universitext, Springer, 2006
[6] Acceleration Methods, Found. Trends Optim., Volume 5 (2021) no. 1-2, pp. 1-245 | DOI
[7] Stochastic mirror descent: Convergence analysis and adaptive variants via the mirror stochastic Polyak stepsize, Transactions on Machine Learning Research (TMLR) (2021)
[8] Polynomial based iteration methods for symmetric linear systems, Society for Industrial and Applied Mathematics, 2011 | DOI
[9] Chebyshev semi-iterative methods, successive overrelaxation iterative methods, and second order Richardson iterative methods, Numer. Math., Volume 3 (1961) no. 1, pp. 157-168 | DOI | Zbl
[10] Super-acceleration with cyclical step-sizes, International Conference on Artificial Intelligence and Statistics, PMLR (2022), pp. 3028-3065
[11] Provable non-accelerations of the heavy-ball method (2023) | arXiv
[12] Cutting Some Slack for SGD with Adaptive Polyak Stepsizes (2022) | arXiv
[13] A survey of nonlinear conjugate gradient methods, Pac. J. Optim., Volume 2 (2006) no. 1, pp. 35-58 | Zbl
[14] Revisiting the Polyak step size (2019) | arXiv
[15] Stochastic Polyak step-size for SGD: An adaptive learning rate for fast convergence, International Conference on Artificial Intelligence and Statistics (AISTATS), PMLR (2021), pp. 1306-1314
[16] Adaptive Gradient Descent without Descent, International Conference on Machine Learning (ICML), JMLR.org, PMLR (2020), pp. 6702-6712
[17] A method of solving a convex programming problem with convergence rate , Sov. Math., Dokl., Volume 27 (1983) no. 2, pp. 372-376 | Zbl
[18] Introductory Lectures on Convex Optimization, Applied Optimization, 87, Springer, 2003 | Zbl
[19] Numerical optimization, Springer, 1999 | DOI
[20] A Hitchhiker’s Guide to Momentum, http://fa.bianp.net/blog/2021/hitchhiker/, 2021 http://fa.bianp.net/blog/2020/polyopt/
[21] Some methods of speeding up the convergence of iteration methods, U.S.S.R. Comput. Math. Math. Phys., Volume 4 (1964) no. 5, pp. 1-17 | DOI | Zbl
[22] Introduction to optimization, Optimization Software New York, 1987
Cited by Sources: