We consider the problem of computing mixed Nash equilibria of two-player zero-sum games with continuous sets of pure strategies and with first-order access to the payoff function. This problem arises for example in game-theory-inspired machine learning applications, such as distributionally-robust learning. In those applications, the strategy sets are high-dimensional and thus methods based on discretisation cannot tractably return high-accuracy solutions. In this paper, we introduce and analyze a particle-based method that enjoys guaranteed local convergence for this problem. This method consists in parametrizing the mixed strategies as atomic measures and applying proximal point updates to both the atoms’ weights and positions. It can be interpreted as an implicit time discretization of the “interacting” Wasserstein–Fisher–Rao gradient flow.
We prove that, under non-degeneracy assumptions, this method converges at an exponential rate to the exact mixed Nash equilibrium from any initialization satisfying a natural notion of closeness to optimality. We illustrate our results with numerical experiments and discuss applications to max-margin and distributionally-robust classification using two-layer neural networks, where our method has a natural interpretation as a simultaneous training of the network’s weights and of the adversarial distribution.
Revised:
Accepted:
Published online:
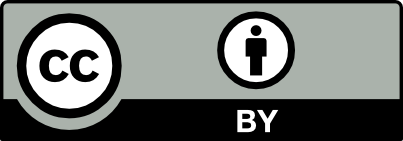
@article{OJMO_2025__6__A1_0, author = {Guillaume Wang and L\'ena{\"\i}c Chizat}, title = {An {Exponentially} {Converging} {Particle} {Method} for the {Mixed} {Nash} {Equilibrium} of {Continuous} {Games}}, journal = {Open Journal of Mathematical Optimization}, eid = {1}, pages = {1--66}, publisher = {Universit\'e de Montpellier}, volume = {6}, year = {2025}, doi = {10.5802/ojmo.37}, language = {en}, url = {https://ojmo.centre-mersenne.org/articles/10.5802/ojmo.37/} }
TY - JOUR AU - Guillaume Wang AU - Lénaïc Chizat TI - An Exponentially Converging Particle Method for the Mixed Nash Equilibrium of Continuous Games JO - Open Journal of Mathematical Optimization PY - 2025 SP - 1 EP - 66 VL - 6 PB - Université de Montpellier UR - https://ojmo.centre-mersenne.org/articles/10.5802/ojmo.37/ DO - 10.5802/ojmo.37 LA - en ID - OJMO_2025__6__A1_0 ER -
%0 Journal Article %A Guillaume Wang %A Lénaïc Chizat %T An Exponentially Converging Particle Method for the Mixed Nash Equilibrium of Continuous Games %J Open Journal of Mathematical Optimization %D 2025 %P 1-66 %V 6 %I Université de Montpellier %U https://ojmo.centre-mersenne.org/articles/10.5802/ojmo.37/ %R 10.5802/ojmo.37 %G en %F OJMO_2025__6__A1_0
Guillaume Wang; Lénaïc Chizat. An Exponentially Converging Particle Method for the Mixed Nash Equilibrium of Continuous Games. Open Journal of Mathematical Optimization, Volume 6 (2025), article no. 1, 66 p. doi : 10.5802/ojmo.37. https://ojmo.centre-mersenne.org/articles/10.5802/ojmo.37/
[1] Reparameterizing mirror descent as gradient descent, Adv. Neural Inf. Process. Syst., Volume 33 (2020), pp. 8430-8439
[2] Breaking the curse of dimensionality with convex neural networks, J. Mach. Learn. Theory, Volume 18 (2017) no. 1, pp. 629-681
[3] Multiplicative weights update in zero-sum games, Proceedings of the 2018 ACM Conference on Economics and Computation (2018), pp. 321-338 | DOI
[4] ORF523: Mirror Descent, part II/II | I’m a bandit, 2013 (Accessed 2022-06-08 https://blogs.princeton.edu/imabandit/2013/04/18/orf523-mirror-descent-part-iiii/)
[5] ORF523: Mirror Prox | I’m a bandit, 2013 (Accessed 2022-06-08 https://blogs.princeton.edu/imabandit/2013/04/23/orf523-mirror-prox/)
[6] Fast policy extragradient methods for competitive games with entropy regularization, Adv. Neural Inf. Process. Syst., Volume 34 (2021), pp. 27952-27964
[7] Convergence rates of gradient methods for convex optimization in the space of measures (2021) | arXiv
[8] Sparse optimization on measures with over-parameterized gradient descent, Math. Program., Volume 194 (2022) no. 1, pp. 487-532 | DOI | MR | Zbl
[9] Unbalanced optimal transport: Dynamic and Kantorovich formulations, J. Funct. Anal., Volume 274 (2018) no. 11, pp. 3090-3123 | DOI | MR | Zbl
[10] Last-Iterate Convergence: Zero-Sum Games and Constrained Min-Max Optimization, 10th innovations in theoretical computer science conference, ITCS 2019, January 10–12, 2019, San Diego, CA, USA (LIPIcs – Leibniz International Proceedings in Informatics), Volume 124, Leibniz Zentrum für Informatik (2019) article no. 27 (18 pages) | MR | Zbl
[11] Efficient methods for structured nonconvex-nonconcave min-max optimization, International Conference on Artificial Intelligence and Statistics, PMLR (2021), pp. 2746-2754
[12] A mean-field analysis of two-player zero-sum games, Adv. Neural Inf. Process. Syst., Volume 33 (2020), pp. 20215-20226
[13] A Variational Inequality Perspective on Generative Adversarial Networks, International Conference on Learning Representations (2018)
[14] A further generalization of the Kakutani fixed point theorem, with application to Nash equilibrium points, Proc. Am. Math. Soc., Volume 3 (1952) no. 1, pp. 170-174 | MR | Zbl
[15] A study of condition numbers for first-order optimization, International Conference on Artificial Intelligence and Statistics, PMLR (2021), pp. 1261-1269
[16] Finding mixed Nash equilibria of generative adversarial networks, International Conference on Machine Learning, PMLR (2019), pp. 2810-2819
[17] Explore aggressively, update conservatively: Stochastic extragradient methods with variable stepsize scaling, Adv. Neural Inf. Process. Syst., Volume 33 (2020), pp. 16223-16234
[18] A Dynamical System View of Langevin-Based Non-Convex Sampling, Advances in Neural Information Processing Systems 36 (NeurIPS 2023) (2023), pp. 41051-41075
[19] Stochastic Approximation Algorithms for Systems of Interacting Particles, Advances in Neural Information Processing Systems 36 (NeurIPS 2023) (2023), pp. 55826-88847
[20] A new optimal transport distance on the space of finite Radon measures, Adv. Differ. Equ., Volume 21 (2016) no. 11/12, pp. 1117-1164 | MR | Zbl
[21] Interaction matters: A note on non-asymptotic local convergence of generative adversarial networks, The 22nd International Conference on Artificial Intelligence and Statistics, PMLR (2019), pp. 907-915
[22] Optimal entropy-transport problems and a new Hellinger–Kantorovich distance between positive measures, Invent. Math., Volume 211 (2018) no. 3, pp. 969-1117 | DOI | MR | Zbl
[23] Two-Scale Gradient Descent Ascent Dynamics Finds Mixed Nash Equilibria of Continuous Games: A Mean-Field Perspective (2022) | arXiv
[24] Provably convergent quasistatic dynamics for mean-field two-player zero-sum games, International Conference on Learning Representations (2021)
[25] Learning in games from a stochastic approximation viewpoint (2022) | arXiv
[26] Optimistic mirror descent in saddle-point problems: Going the extra (gradient) mile, International Conference on Learning Representations (2018)
[27] Cycles in adversarial regularized learning, Proceedings of the Twenty-Ninth Annual ACM-SIAM Symposium on Discrete Algorithms, Association for Computing Machinery (2018), pp. 2703-2717 | Zbl
[28] Data-driven distributionally robust optimization using the Wasserstein metric: Performance guarantees and tractable reformulations, Math. Program., Volume 171 (2018) no. 1, pp. 115-166 | DOI | MR | Zbl
[29] A unified analysis of extra-gradient and optimistic gradient methods for saddle point problems: Proximal point approach, International Conference on Artificial Intelligence and Statistics, PMLR (2020), pp. 1497-1507
[30] Prox-method with rate of convergence O (1/t) for variational inequalities with Lipschitz continuous monotone operators and smooth convex-concave saddle point problems, SIAM J. Optim., Volume 15 (2004) no. 1, pp. 229-251 | DOI | MR | Zbl
[31] Approximation theory of the MLP model in neural networks, Acta Numer., Volume 8 (1999), pp. 143-195 | DOI | Zbl
[32] Euclidean, metric, and Wasserstein gradient flows: an overview, Bull. Math. Sci., Volume 7 (2017) no. 1, pp. 87-154 | DOI | MR | Zbl
[33] On general minimax theorems, Pac. J. Math., Volume 8 (1958) no. 1, pp. 171-176 | DOI | MR | Zbl
[34] Separable and low-rank continuous games, Int. J. Game Theory, Volume 37 (2008) no. 4, pp. 475-504 | DOI | MR | Zbl
[35] On linear convergence of iterative methods for the variational inequality problem, J. Comput. Appl. Math., Volume 60 (1995) no. 1-2, pp. 237-252 | DOI | MR | Zbl
[36] Local convergence of gradient methods for min-max games: partial curvature generically suffices, Advances in Neural Information Processing Systems 36 (NeurIPS 2023) (2023), pp. 60841-60852
[37] Linear Last-iterate Convergence in Constrained Saddle-point Optimization, International Conference on Learning Representations (2020)
Cited by Sources: