The M-P (Moore–Penrose) pseudoinverse has as a key application the computation of least-squares solutions of inconsistent systems of linear equations. Irrespective of whether a given input matrix is sparse, its M-P pseudoinverse can be dense, potentially leading to high computational burden, especially when we are dealing with high-dimensional matrices. The M-P pseudoinverse is uniquely characterized by four properties, but only two of them need to be satisfied for the computation of least-squares solutions. Fampa and Lee (2018) and Xu, Fampa, Lee, and Ponte (2019) propose local-search procedures to construct sparse block-structured generalized inverses that satisfy the two key M-P properties, plus one more (the so-called reflexive property). That additional M-P property is equivalent to imposing a minimum-rank condition on the generalized inverse. (Vector) 1-norm minimization is used to induce sparsity and, importantly, to keep the magnitudes of entries under control for the generalized-inverses constructed. Here, we investigate the trade-off between low 1-norm and low rank for generalized inverses that can be used in the computation of least-squares solutions. We propose several algorithmic approaches that start from a -norm minimizing generalized inverse that satisfies the two key M-P properties, and gradually decrease its rank, by iteratively imposing the reflexive property. The algorithms iterate until the generalized inverse has the least possible rank. During the iterations, we produce intermediate solutions, trading off low 1-norm (and typically high sparsity) against low rank.
Revised:
Accepted:
Published online:
Marcia Fampa 1; Jon Lee 2; Gabriel Ponte 1
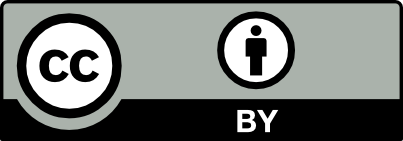
@article{OJMO_2021__2__A4_0, author = {Marcia Fampa and Jon Lee and Gabriel Ponte}, title = {Trading off 1-norm and sparsity against rank for linear models using mathematical optimization: 1-norm minimizing partially reflexive ah-symmetric generalized inverses}, journal = {Open Journal of Mathematical Optimization}, eid = {4}, pages = {1--14}, publisher = {Universit\'e de Montpellier}, volume = {2}, year = {2021}, doi = {10.5802/ojmo.6}, language = {en}, url = {https://ojmo.centre-mersenne.org/articles/10.5802/ojmo.6/} }
TY - JOUR AU - Marcia Fampa AU - Jon Lee AU - Gabriel Ponte TI - Trading off 1-norm and sparsity against rank for linear models using mathematical optimization: 1-norm minimizing partially reflexive ah-symmetric generalized inverses JO - Open Journal of Mathematical Optimization PY - 2021 SP - 1 EP - 14 VL - 2 PB - Université de Montpellier UR - https://ojmo.centre-mersenne.org/articles/10.5802/ojmo.6/ DO - 10.5802/ojmo.6 LA - en ID - OJMO_2021__2__A4_0 ER -
%0 Journal Article %A Marcia Fampa %A Jon Lee %A Gabriel Ponte %T Trading off 1-norm and sparsity against rank for linear models using mathematical optimization: 1-norm minimizing partially reflexive ah-symmetric generalized inverses %J Open Journal of Mathematical Optimization %D 2021 %P 1-14 %V 2 %I Université de Montpellier %U https://ojmo.centre-mersenne.org/articles/10.5802/ojmo.6/ %R 10.5802/ojmo.6 %G en %F OJMO_2021__2__A4_0
Marcia Fampa; Jon Lee; Gabriel Ponte. Trading off 1-norm and sparsity against rank for linear models using mathematical optimization: 1-norm minimizing partially reflexive ah-symmetric generalized inverses. Open Journal of Mathematical Optimization, Volume 2 (2021), article no. 4, 14 p. doi : 10.5802/ojmo.6. https://ojmo.centre-mersenne.org/articles/10.5802/ojmo.6/
[1] Rank-sparsity incoherence for matrix decomposition, SIAM J. Optim., Volume 21 (2011) no. 2, pp. 572-596 | DOI | MR | Zbl
[2] Beyond Moore-Penrose part I: generalized inverses that minimize matrix norms (2017) (http://arxiv.org/abs/1706.08349) | Zbl
[3] On sparse reflexive generalized inverses, Oper. Res. Lett., Volume 46 (2018) no. 6, pp. 605-610 | DOI | MR | Zbl
[4] Experimental analysis of local search for sparse reflexive generalized inverses (2019) (https://arxiv.org/abs/2001.03732v1)
[5] Sparse pseudoinverses via LP and SDP relaxations of Moore-Penrose, CLAIO 2016 (2016), pp. 343-350
[6] Matrix Computations (3rd Ed.), Johns Hopkins University Press, 1996 | Zbl
[7] Iterative reweighted algorithms for matrix rank minimization, The Journal of Machine Learning Research, Volume 13 (2012), pp. 3441-3473 | MR | Zbl
[8] A generalized inverse for matrices, Proc. Camb. Philos. Soc., Volume 51 (1955), pp. 406-413 | DOI
[9] Guaranteed Minimum-Rank Solutions of Linear Matrix Equations via Nuclear Norm Minimization, SIAM Rev., Volume 52 (2010) no. 3, pp. 471-501 | DOI | MR | Zbl
[10] Contributions to the theory, computation and application of generalized inverses, Ph. D. Thesis, University of North Carolina, Raleigh, N.C. (1964) (https://www4.stat.ncsu.edu/~boos/library/mimeo.archive/ISMS_1964_392.pdf) | MR
[11] Approximate 1-norm minimization and minimum-rank structured sparsity for various generalized inverses via local search (2021) (to appear in SIAM J. Optim.)
Cited by Sources: