Iterative optimization algorithms depend on access to information about the objective function. In a differentiable programming framework, this information, such as gradients, can be automatically derived from the computational graph. We explore how nonlinear control algorithms, often employing linear and/or quadratic approximations, can be effectively cast within this framework. Our approach illuminates shared components and differences between gradient descent, Gauss–Newton, Newton, and differential dynamic programming methods in the context of discrete time nonlinear control. Furthermore, we present line-search strategies and regularized variants of these algorithms, along with a comprehensive analysis of their computational complexities. We study the performance of the aforementioned algorithms on various nonlinear control benchmarks, including autonomous car racing simulations using a simplified car model. All implementations are publicly available in a package coded in a differentiable programming language.
Revised:
Accepted:
Published online:
DOI: 10.5802/ojmo.32
Vincent Roulet 1; Siddhartha Srinivasa 2; Maryam Fazel 3; Zaid Harchaoui 4
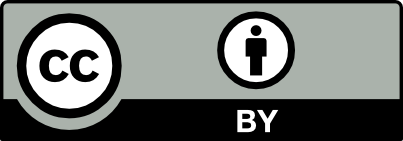
@article{OJMO_2024__5__A9_0, author = {Vincent Roulet and Siddhartha Srinivasa and Maryam Fazel and Zaid Harchaoui}, title = {Iterative {Linear} {Quadratic} {Optimization} for {Nonlinear} {Control:} {Differentiable} {Programming} {Algorithmic} {Templates}}, journal = {Open Journal of Mathematical Optimization}, eid = {8}, pages = {1--63}, publisher = {Universit\'e de Montpellier}, volume = {5}, year = {2024}, doi = {10.5802/ojmo.32}, mrnumber = {4822314}, language = {en}, url = {https://ojmo.centre-mersenne.org/articles/10.5802/ojmo.32/} }
TY - JOUR AU - Vincent Roulet AU - Siddhartha Srinivasa AU - Maryam Fazel AU - Zaid Harchaoui TI - Iterative Linear Quadratic Optimization for Nonlinear Control: Differentiable Programming Algorithmic Templates JO - Open Journal of Mathematical Optimization PY - 2024 SP - 1 EP - 63 VL - 5 PB - Université de Montpellier UR - https://ojmo.centre-mersenne.org/articles/10.5802/ojmo.32/ DO - 10.5802/ojmo.32 LA - en ID - OJMO_2024__5__A9_0 ER -
%0 Journal Article %A Vincent Roulet %A Siddhartha Srinivasa %A Maryam Fazel %A Zaid Harchaoui %T Iterative Linear Quadratic Optimization for Nonlinear Control: Differentiable Programming Algorithmic Templates %J Open Journal of Mathematical Optimization %D 2024 %P 1-63 %V 5 %I Université de Montpellier %U https://ojmo.centre-mersenne.org/articles/10.5802/ojmo.32/ %R 10.5802/ojmo.32 %G en %F OJMO_2024__5__A9_0
Vincent Roulet; Siddhartha Srinivasa; Maryam Fazel; Zaid Harchaoui. Iterative Linear Quadratic Optimization for Nonlinear Control: Differentiable Programming Algorithmic Templates. Open Journal of Mathematical Optimization, Volume 5 (2024), article no. 8, 63 p. doi : 10.5802/ojmo.32. https://ojmo.centre-mersenne.org/articles/10.5802/ojmo.32/
[1] TensorFlow: Large-Scale Machine Learning on Heterogeneous Systems, 2015 (https://tensorflow.org/)
[2] CasADi – A software framework for nonlinear optimization and optimal control, Math. Program. Comput., Volume 11 (2019), pp. 1-36 | DOI | MR | Zbl
[3] PROX-QP: Yet another quadratic programming solver for robotics and beyond (2022) (RSS 2022-Robotics: Science and Systems)
[4] The complexity of partial derivatives, Theor. Comput. Sci., Volume 22 (1983) no. 3, pp. 317-330 | DOI | MR | Zbl
[5] Automatic differentiation in machine learning: a survey, J. Mach. Learn. Res., Volume 18 (2018), 153, 43 pages | MR | Zbl
[6] Introduction to the mathematical theory of control processes. Vol. II: Nonlinear processes, Mathematics in Science and Engineering, 40-II, Academic Press Inc., 1971 | MR
[7] Practical methods for optimal control and estimation using nonlinear programming, Society for Industrial and Applied Mathematics, 2010 | DOI | MR
[8] A multiple shooting algorithm for direct solution of optimal control problems, IFAC Proceedings Volumes, Volume 17 (1984) no. 2, pp. 1603-1608 | DOI
[9] A mathematical model for automatic differentiation in machine learning, Proceedings of the 34rd International Conference on Neural Information Processing Systems, Curran Associates, Inc. (2020)
[10] Semidefinite programming relaxations of non-convex problems in control and combinatorial optimization, Communications, Computation, Control, and Signal Processing, Springer, 1997, pp. 279-287 | DOI | MR
[11] Convex optimization, Cambridge University Press, 2004 | DOI | MR
[12] JAX: composable transformations of Python+NumPy programs, 2018 (version 0.3.13, https://github.com/google/jax)
[13] Pyomo–optimization modeling in python, Springer Optimization and Its Applications, 67, Springer, 2021 | MR | Zbl
[14] Fast direct multiple shooting algorithms for optimal robot control, Fast motions in biomechanics and robotics: optimization and feedback control (Lecture Notes in Control and Information Sciences), Volume 340, Springer, 2006, pp. 65-93 | DOI | Zbl
[15] Efficient numerical methods for nonlinear MPC and moving horizon estimation, Nonlinear model predictive control: towards new challenging applications (Lecture Notes in Control and Information Sciences), Volume 384, Springer, 2009, pp. 391-417 | DOI | Zbl
[16] Efficient dynamic programming implementations of Newton’s method for unconstrained optimal control problems, J. Optim. Theory Appl., Volume 63 (1989) no. 1, pp. 23-38 | DOI | MR | Zbl
[17] JuMP: A modeling language for mathematical optimization, SIAM Rev., Volume 59 (2017) no. 2, pp. 295-320 | DOI | MR | Zbl
[18] An efficient optimal planning and control framework for quadrupedal locomotion, 2017 IEEE International Conference on Robotics and Automation (ICRA), IEEE (2017), pp. 93-100 | DOI
[19] A parallel quadratic programming method for dynamic optimization problems, Math. Program. Comput., Volume 7 (2015) no. 3, pp. 289-329 | DOI | MR | Zbl
[20] A family of iterative Gauss-Newton shooting methods for nonlinear optimal control, 2018 IEEE/RSJ International Conference on Intelligent Robots and Systems (IROS), IEEE (2018), pp. 1-9
[21] Automatic differentiation and iterative processes, Optim. Methods Softw., Volume 1 (1992) no. 1, pp. 13-21 | DOI
[22] SNOPT: An SQP algorithm for large-scale constrained optimization, SIAM Rev., Volume 47 (2005) no. 1, pp. 99-131 | DOI | MR | Zbl
[23] Deep learning, MIT Press, 2016 | MR
[24] Evaluating derivatives: principles and techniques of algorithmic differentiation, Society for Industrial and Applied Mathematics, 2008 | DOI | MR
[25] A quadratically convergent inexact SQP method for optimal control of differential algebraic equations, Optim. Control Appl. Methods, Volume 34 (2013) no. 4, pp. 396-414 | DOI | Zbl
[26] Differential Dynamic Programming, Elsevier, 1970 | MR
[27] Proxddp: Proximal constrained trajectory optimization (2023)
[28] Information theoretic regret bounds for online nonlinear control, Adv. Neural Inf. Process. Syst., Volume 33 (2020), pp. 15312-15325
[29] A theoretical framework for back-propagation, 1988 Connectionist Models Summer School, CMU, Pittsburg, PA (1988)
[30] Iterative linearization methods for approximately optimal control and estimation of non-linear stochastic system, Int. J. Control, Volume 80 (2007) no. 9, pp. 1439-1453 | DOI | MR | Zbl
[31] Convergence in unconstrained discrete-time differential dynamic programming, IEEE Trans. Autom. Control, Volume 36 (1991) no. 6, pp. 692-706 | DOI | MR | Zbl
[32] Advantages of differential dynamic programming over Newton’s method for discrete-time optimal control problems (1992) (Technical report)
[33] Optimization-based autonomous racing of 1: 43 scale RC cars, Optim. Control Appl. Methods, Volume 36 (2015) no. 5, pp. 628-647 | DOI | MR | Zbl
[34] Generalized Solutions of Hamilton-Jacobi Equations, Pitman, 1982 | MR
[35] Modeling of inverted pendulum system with gravitational search algorithm optimized controller, Ain Shams Engineering Journal, Volume 10 (2019) no. 1, pp. 129-149 | DOI
[36] First-order strong variation algorithms for optimal control, J. Optim. Theory Appl., Volume 16 (1975) no. 3, pp. 277-301 | DOI | MR
[37] Survey of sequential convex programming and generalized Gauss–Newton methods, ESAIM, Proc. Surv., Volume 71 (2021), pp. 64-88 | DOI | MR | Zbl
[38] Differential dynamic programming and Newton’s method for discrete optimal control problems, J. Optim. Theory Appl., Volume 43 (1984) no. 3, pp. 395-414 | DOI | MR | Zbl
[39] Lectures on convex optimization, Springer, 2018 | DOI | MR
[40] Accelerating Second-Order Differential Dynamic Programming for Rigid-Body Systems, IEEE Robotics and Automation Letters, Volume 6 (2021) no. 4, pp. 7659-7666 | DOI
[41] Numerical optimization, Springer, 2006 | MR
[42] Differential dynamic programming and Newton’s method, Int. J. Control, Volume 47 (1988) no. 5, pp. 1539-1553 | DOI | MR | Zbl
[43] PyTorch: An Imperative Style, High-Performance Deep Learning Library, Proceedings of the 33rd International Conference on Neural Information Processing Systems, Curran Associates, Inc. (2019), pp. 8026-8037
[44] Computational methods in optimization: a unified approach, Mathematics in Science and Engineering, 77, Academic Press Inc., 1971 | MR
[45] Application of interior-point methods to model predictive control, J. Optim. Theory Appl., Volume 99 (1998) no. 3, pp. 723-757 | MR | Zbl
[46] A tour of reinforcement learning: The view from continuous control, Annual Review of Control, Robotics, and Autonomous Systems, Volume 2 (2019), pp. 253-279 | DOI
[47] Iterative linearized control: stable algorithms and complexity guarantees, Proceedings of the 36th International Conference on Machine Learning (Proceedings of Machine Learning Research), Volume 97, JMLR (2019), pp. 5518-5527
[48] Learning representations by back-propagating errors, Nature, Volume 323 (1986) no. 6088, pp. 533-536 | DOI | Zbl
[49] Making the world differentiable: on using self supervised fully recurrent neural networks for dynamic reinforcement learning and planning in non-stationary environments (1990) (Technical report)
[50] An efficient sequential linear quadratic algorithm for solving nonlinear optimal control problems, Proceedings of the 2005 American Control Conference, IEEE (2005), pp. 2275-2280 | DOI
[51] Graphical Newton (2015) | arXiv
[52] Receding horizon differential dynamic programming, Adv. Neural Inf. Process. Syst., Volume 20 (2007)
[53] Synthesis and stabilization of complex behaviors through online trajectory optimization, 2012 IEEE/RSJ International Conference on Intelligent Robots and Systems, IEEE (2012), pp. 4906-4913
[54] Control-limited differential dynamic programming, 2014 IEEE International Conference on Robotics and Automation (ICRA), IEEE (2014), pp. 1168-1175 | DOI
[55] MuJoCo: A physics engine for model-based control, International Conference on Intelligent Robots and Systems (IROS), IEEE (2012), pp. 5026-5033
[56] acados — a modular open-source framework for fast embedded optimal control, Math. Program. Comput., Volume 14 (2022), pp. 147-183 | DOI | MR | Zbl
[57] Exploiting convexity in direct optimal control: a sequential convex quadratic programming method, 2016 IEEE 55th Conference on Decision and Control (CDC), IEEE (2016), pp. 1099-1104 | DOI
[58] Numerical solution of optimal control problems by direct collocation, Springer, 1993
[59] On the implementation of an interior-point filter line-search algorithm for large-scale nonlinear programming, Math. Program., Volume 106 (2006), pp. 25-57 | DOI | MR | Zbl
[60] The Roots of Backpropagation: From Ordered Derivatives to Neural Networks and Political Forecasting, Wiley-Interscience, 1994
[61] Solution of discrete-time optimal control problems on parallel computers, Parallel Comput., Volume 16 (1990) no. 2-3, pp. 221-237 | DOI | MR | Zbl
[62] Partitioned dynamic programming for optimal control, SIAM J. Optim., Volume 1 (1991) no. 4, pp. 620-642 | DOI | MR | Zbl
[63] Structured interior point methods for optimal control, Proceedings of the 30th IEEE Conference on Decision and Control, IEEE (1991), pp. 1711-1716 | DOI
[64] Interior point methods for optimal control of discrete time systems, J. Optim. Theory Appl., Volume 77 (1993) no. 1, pp. 161-187 | DOI | MR | Zbl
[65] Dive into Deep Learning, Cambridge University Press, 2023
Cited by Sources: