Frank and Wolfe’s celebrated conditional gradient method is a well-known tool for solving smooth optimization problems for which minimizing a linear function over the feasible set is computationally cheap. However, when the objective function is nonsmooth, the method may fail to compute a stationary point. In this work, we show that the Frank–Wolfe algorithm can be employed to compute Clarke-stationary points for nonconvex and nonsmooth optimization problems consisting of minimizing upper- functions over convex and compact sets. Furthermore, under more restrictive assumptions, we propose a new algorithm variant with stronger stationarity guarantees, namely directional stationarity and even local optimality.
Revised:
Accepted:
Published online:
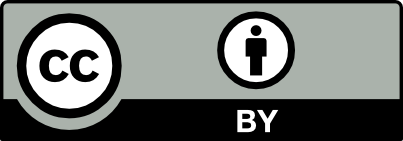
@article{OJMO_2023__4__A2_0, author = {Welington de Oliveira}, title = {Short {Paper} - {A} note on the {Frank{\textendash}Wolfe} algorithm for a class of nonconvex and nonsmooth optimization problems}, journal = {Open Journal of Mathematical Optimization}, eid = {2}, pages = {1--10}, publisher = {Universit\'e de Montpellier}, volume = {4}, year = {2023}, doi = {10.5802/ojmo.21}, language = {en}, url = {https://ojmo.centre-mersenne.org/articles/10.5802/ojmo.21/} }
TY - JOUR AU - Welington de Oliveira TI - Short Paper - A note on the Frank–Wolfe algorithm for a class of nonconvex and nonsmooth optimization problems JO - Open Journal of Mathematical Optimization PY - 2023 SP - 1 EP - 10 VL - 4 PB - Université de Montpellier UR - https://ojmo.centre-mersenne.org/articles/10.5802/ojmo.21/ DO - 10.5802/ojmo.21 LA - en ID - OJMO_2023__4__A2_0 ER -
%0 Journal Article %A Welington de Oliveira %T Short Paper - A note on the Frank–Wolfe algorithm for a class of nonconvex and nonsmooth optimization problems %J Open Journal of Mathematical Optimization %D 2023 %P 1-10 %V 4 %I Université de Montpellier %U https://ojmo.centre-mersenne.org/articles/10.5802/ojmo.21/ %R 10.5802/ojmo.21 %G en %F OJMO_2023__4__A2_0
Welington de Oliveira. Short Paper - A note on the Frank–Wolfe algorithm for a class of nonconvex and nonsmooth optimization problems. Open Journal of Mathematical Optimization, Volume 4 (2023), article no. 2, 10 p. doi : 10.5802/ojmo.21. https://ojmo.centre-mersenne.org/articles/10.5802/ojmo.21/
[1] Duality between subgradient and conditional gradient methods, SIAM J. Optim., Volume 26 (2015) no. 1, pp. 115-129 | DOI | Zbl
[2] First-Order Methods in Optimization, MOS-SIAM Series on Optimization, 25, Society for Industrial and Applied Mathematics, 2017 | DOI | Numdam
[3] On the convergence to stationary points of deterministic and randomized feasible descent directions methods, SIAM J. Optim., Volume 30 (2020) no. 1, pp. 56-79 | DOI | Zbl
[4] The alternating descent conditional gradient method for sparse inverse problems, SIAM J. Optim., Volume 27 (2017) no. 2, pp. 616-639 | DOI | Zbl
[5] Optimisation and Nonsmooth Analysis, Classics in Applied Mathematics, 5, Society for Industrial and Applied Mathematics, 1990 | DOI
[6] Boosting Frank–Wolfe by chasing gradients, Proceedings of the 37th International Conference on Machine Learning (Proceedings of Machine Learning Research), Volume 119, PMLR, 2020, pp. 2111-2121
[7] Filling the gap between lower- and lower- functions, J. Convex Anal., Volume 12 (2005) no. 2, pp. 315-329 | Zbl
[8] The ABC of DC programming, Set-Valued Var. Anal., Volume 28 (2020) no. 4, pp. 679-706 | DOI | Zbl
[9] The sliding Frank–Wolfe algorithm and its application to super-resolution microscopy, Inverse Probl., Volume 36 (2020) no. 1, 014001, 42 pages | Zbl
[10] Randomized smoothing for stochastic optimization, SIAM J. Optim., Volume 22 (2012) no. 2, pp. 674-701 | DOI | Zbl
[11] An algorithm for quadratic programming, Nav. Res. Logist. Q., Volume 3 (1956) no. 1-2, pp. 95-110 | DOI
[12] Revisiting Frank–Wolfe: Projection-free sparse convex optimization, Proceedings of the 30th International Conference on Machine Learning (Proceedings of Machine Learning Research), Volume 28, PMLR, 2013, pp. 427-435
[13] Regularized Frank–Wolfe for dense CRFs: Generalizing mean field and beyond, Advances in Neural Information Processing Systems, Volume 34, Neural Information Processing Systems, 2021, pp. 1453-1467
[14] A minimization method for the sum of a convex function and a continuously differentiable function, J. Optim. Theory Appl., Volume 33 (1981) no. 1, pp. 9-23 | DOI
[15] Complexity bounds for primal-dual methods minimizing the model of objective function, Math. Program., Volume 171 (2018) no. 1-2, pp. 311-330 | DOI | Zbl
[16] Minding the gaps for block Frank–Wolfe optimization of structured SVMs, Proceedings of the 33rd International Conference of Machine Learning, ICML, 2016
[17] Computing B-stationary points of nonsmooth DC programs, Math. Oper. Res., Volume 42 (2017) no. 1, pp. 95-118 | DOI | Zbl
[18] Linearly convergent Frank–Wolfe with backtracking line-search, Proceedings of the Twenty Third International Conference on Artificial Intelligence and Statistics (Proceedings of Machine Learning Research), Volume 108, PMLR, 2020, pp. 1-10
[19] A deterministic nonsmooth Frank–Wolfe algorithm with coreset guarantees, INFORMS J. Optim., Volume 1 (2019) no. 2, pp. 120-142 | DOI
[20] Favorable classes of lipschitz continuous functions in subgradient optimization, Progress in Nondifferentiable Optimization (IIASA Collaborative Proceedings Series), International Institute of Applied Systems Analysis, 1982, pp. 125-144 | Zbl
[21] Generalized subgradients in mathematical programming, Mathematical Programming The State of the Art, XIth International Symposium on Mathematical Programming, Bonn, Germany, August 23-27, Springer, 1982, pp. 23-27
[22] Variational Analysis, Grundlehren der Mathematischen Wissenschaften, 317, Springer, 2009
[23] Submonotone subdifferentials of Lipschitz functions, Trans. Am. Math. Soc., Volume 264 (1981), pp. 77-89 | DOI | Zbl
[24] Projection efficient subgradient method and optimal nonsmooth Frank–Wolfe method, Advances in Neural Information Processing Systems 33, Curran Associates, Inc., 2020, pp. 12211-12224
[25] Extension of the Frank–Wolfe algorithm to concave nondifferentiable objective functions, J. Optim. Theory Appl., Volume 78 (1993) no. 2, pp. 283-301 | DOI | Zbl
Cited by Sources: