It was recently shown [6, 8] that “properly built” linear and polyhedral estimates nearly attain minimax accuracy bounds in the problem of recovery of unknown signal from noisy observations of linear images of the signal when the signal set is an ellitope. However, design of nearly optimal estimates relies upon solving semidefinite optimization problems with matrix variables, what puts the synthesis of such estimates beyond the reach of the standard Interior Point algorithms of semidefinite optimization even for moderate size recovery problems. Our goal is to develop First Order Optimization algorithms for the computationally efficient design of linear and polyhedral estimates. In this paper we (a) explain how to eliminate matrix variables, thus reducing dramatically the design dimension when passing from Interior Point to First Order optimization algorithms and (b) develop and analyse a dedicated algorithm of the latter type — Composite Truncated Level method.
Revised:
Accepted:
Published online:
DOI: 10.5802/ojmo.35
Yannis Bekri 1; Anatoli Juditsky 1; Arkadi Nemirovski 2
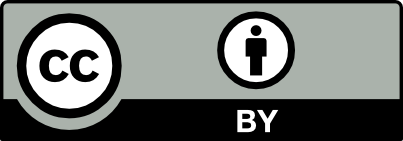
@article{OJMO_2024__5__A8_0, author = {Yannis Bekri and Anatoli Juditsky and Arkadi Nemirovski}, title = {First order algorithms for computing linear and polyhedral estimates}, journal = {Open Journal of Mathematical Optimization}, eid = {7}, pages = {1--15}, publisher = {Universit\'e de Montpellier}, volume = {5}, year = {2024}, doi = {10.5802/ojmo.35}, mrnumber = {4822313}, language = {en}, url = {https://ojmo.centre-mersenne.org/articles/10.5802/ojmo.35/} }
TY - JOUR AU - Yannis Bekri AU - Anatoli Juditsky AU - Arkadi Nemirovski TI - First order algorithms for computing linear and polyhedral estimates JO - Open Journal of Mathematical Optimization PY - 2024 SP - 1 EP - 15 VL - 5 PB - Université de Montpellier UR - https://ojmo.centre-mersenne.org/articles/10.5802/ojmo.35/ DO - 10.5802/ojmo.35 LA - en ID - OJMO_2024__5__A8_0 ER -
%0 Journal Article %A Yannis Bekri %A Anatoli Juditsky %A Arkadi Nemirovski %T First order algorithms for computing linear and polyhedral estimates %J Open Journal of Mathematical Optimization %D 2024 %P 1-15 %V 5 %I Université de Montpellier %U https://ojmo.centre-mersenne.org/articles/10.5802/ojmo.35/ %R 10.5802/ojmo.35 %G en %F OJMO_2024__5__A8_0
Yannis Bekri; Anatoli Juditsky; Arkadi Nemirovski. First order algorithms for computing linear and polyhedral estimates. Open Journal of Mathematical Optimization, Volume 5 (2024), article no. 7, 15 p. doi : 10.5802/ojmo.35. https://ojmo.centre-mersenne.org/articles/10.5802/ojmo.35/
[1] On robust recovery of signals from indirect observations (2023) | arXiv
[2] Lectures on modern convex optimization: analysis, algorithms, and engineering applications, MPS/SIAM Series on Optimization, 2, Society for Industrial and Applied Mathematics, 2001 | DOI | MR | Zbl
[3] Non-euclidean restricted memory level method for large-scale convex optimization, Math. Program., Volume 102 (2005) no. 3, pp. 407-456 | DOI | MR | Zbl
[4] The CVX Users’ Guide. Release 2.1, 2014 (https://web.cvxr.com/cvx/doc/CVX.pdf)
[5] A tail inequality for quadratic forms of subgaussian random vectors, Electron. Commun. Probab., Volume 17 (2012), 52, 6 pages | MR | Zbl
[6] Near-optimality of linear recovery from indirect observations, Math. Stat. Learn., Volume 1 (2018) no. 2, pp. 171-225 | DOI | MR | Zbl
[7] Near-optimality of linear recovery in gaussian observation scheme under -loss, Ann. Stat., Volume 46 (2018) no. 2, pp. 1603-1629 | MR | Zbl
[8] On polyhedral estimation of signals via indirect observations, Electron. J. Stat., Volume 14 (2020) no. 1, p. 458--502 | MR | Zbl
[9] Statistical Inference via Convex Optimization, Princeton Series in Applied Mathematics, Princeton University Press, 2020, xxiv+631 pages | MR
[10] Proximal level bundle methods for convex nondifferentiable optimization, saddle-point problems and variational inequalities, Math. Program., Volume 69 (1995) no. 1, pp. 89-109 | DOI | MR | Zbl
[11] On first-order algorithms for /nuclear norm minimization, Acta Numer., Volume 22 (2013), pp. 509-575 | DOI | MR | Zbl
[12] Hanson–Wright inequality and sub-Gaussian concentration, Electron. Commun. Probab., Volume 18 (2013), 82, 9 pages | MR | Zbl
[13] Sharp deviation bounds for quadratic forms, Math. Methods Stat., Volume 22 (2013) no. 2, pp. 100-113 | DOI | MR | Zbl
Cited by Sources: