Probability functions measure the degree of satisfaction of certain constraints that are impacted by decisions and uncertainty. Such functions appear in probability or chance constraints ensuring that the degree of satisfaction is sufficiently high. These constraints have become a very popular modelling tool and are indeed intuitively easy to understand. Optimization problems involving probabilistic constraints have thus arisen in many sectors of the industry, such as in the energy sector. Finding an efficient solution methodology is important and first order information of probability functions play a key role therein. In this work we are motivated by probability functions measuring the degree of satisfaction of a potentially heterogenous family of constraints. We suggest a framework wherein each individual such constraint can be analyzed structurally. Our framework then allows us to establish formulae for the generalized subdifferential of the probability function itself. In particular we formally establish a (sub)-gradient formulæ for probability functions depending on a family of non-convex quadratic inequalities. The latter situation is relevant for gas-network applications.
Revised:
Accepted:
Published online:
Keywords: Stochastic optimization, probabilistic constraints, chance constraints, generalized gradients
Wim van Ackooij 1; Pedro Pérez-Aros 2
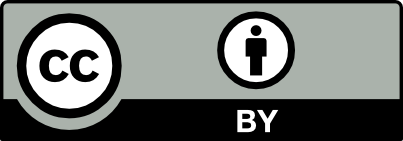
@article{OJMO_2021__2__A7_0, author = {Wim van Ackooij and Pedro P\'erez-Aros}, title = {Gradient formulae for probability functions depending on a heterogenous family of constraints}, journal = {Open Journal of Mathematical Optimization}, eid = {7}, pages = {1--29}, publisher = {Universit\'e de Montpellier}, volume = {2}, year = {2021}, doi = {10.5802/ojmo.9}, language = {en}, url = {https://ojmo.centre-mersenne.org/articles/10.5802/ojmo.9/} }
TY - JOUR AU - Wim van Ackooij AU - Pedro Pérez-Aros TI - Gradient formulae for probability functions depending on a heterogenous family of constraints JO - Open Journal of Mathematical Optimization PY - 2021 SP - 1 EP - 29 VL - 2 PB - Université de Montpellier UR - https://ojmo.centre-mersenne.org/articles/10.5802/ojmo.9/ DO - 10.5802/ojmo.9 LA - en ID - OJMO_2021__2__A7_0 ER -
%0 Journal Article %A Wim van Ackooij %A Pedro Pérez-Aros %T Gradient formulae for probability functions depending on a heterogenous family of constraints %J Open Journal of Mathematical Optimization %D 2021 %P 1-29 %V 2 %I Université de Montpellier %U https://ojmo.centre-mersenne.org/articles/10.5802/ojmo.9/ %R 10.5802/ojmo.9 %G en %F OJMO_2021__2__A7_0
Wim van Ackooij; Pedro Pérez-Aros. Gradient formulae for probability functions depending on a heterogenous family of constraints. Open Journal of Mathematical Optimization, Volume 2 (2021), article no. 7, 29 p. doi : 10.5802/ojmo.9. https://ojmo.centre-mersenne.org/articles/10.5802/ojmo.9/
[1] Joint model of probabilistic (probust) constraints with application to gas network optimization, Vietnam J. Math., Volume Online (2020), pp. 1-34 | DOI
[2] Measure theory. Vol. I and II, Springer, 2007, xviii+500 pp. and xiv+575 pages | DOI | Zbl
[3] Probabilistic constraints via SQP solver: Application to a renewable energy management problem, Comput. Manag. Sci., Volume 12 (2015) no. 3, pp. 435-459 | DOI | MR | Zbl
[4] A non-linear regression model for mid-term load forecasting and improvements in seasonality, 2005 (PSCC 2005 Luik)
[5] Optimisation and Nonsmooth Analysis, Classics in Applied Mathematics, Society for Industrial and Applied Mathematics, 1987, 320 pages | DOI
[6] Measure theory, Birkhäuser Advanced Texts. Basler Lehrbücher, Birkhäuser, 2013, xxi+457 pages | DOI | MR | Zbl
[7] Optimisation models with probabilistic constraints, Lectures on Stochastic Programming. Modeling and Theory (MPS/SIAM Series on Optimization), Volume 9, Society for Industrial and Applied Mathematics, 2009, pp. 87-154 | DOI
[8] Maß und Integrationstheorie, Springer-Lehrbuch, Springer, 2011, 451 pages | DOI | Zbl
[9] Symmetric multivariate and related distributions, Monographs on Statistics and Applied Probability, 36, Chapman & Hall, 1990, x+220 pages | MR | Zbl
[10] Asymptotic formulas for the derivatives of probability functions and their Monte Carlo estimations, Eur. J. Oper. Res., Volume 198 (2009), pp. 848-858 | DOI | MR | Zbl
[11] Numerical computation of multivariate normal probabilities, J. Comput. Graph. Stat., Volume 1 (1992), pp. 141-149
[12] On the quantification of nomination feasibility in stationary gas networks with random loads, Math. Methods Oper. Res., Volume 84 (2016) no. 2, pp. 427-457 | DOI | MR | Zbl
[13] Subdifferential characterization of continuous probability functions under Gaussian distribution, Math. Program., Volume 174 (2019) no. 1-2, pp. 167-194 | DOI | Zbl
[14] On probabilistic capacity maximization in a stationary gas network, Optimization, Volume 69 (2020) no. 3, pp. 575-604 | DOI | MR | Zbl
[15] Optimierungsprobleme mit Wahrscheinlichkeitsrestriktionen: Modelle, Struktur, Numerik, 2016
[16] Lipschitz and differentiability properties of quasi-concave and singular normal distribution functions, Ann. Oper. Res., Volume 177 (2010), pp. 115-125 | DOI | MR | Zbl
[17] Differentiability of Probability function, Stochastic Anal. Appl., Volume 16 (1998), pp. 1101-1128 | DOI | MR | Zbl
[18] Tail Conditional Expectations for Elliptical distributions, N. Am. Actuar. J., Volume 7 (2003) no. 4, pp. 55-71 | DOI | MR | Zbl
[19] Differentiation formulas for probability functions: The transformation method, Math. Program., Volume 75 (1996) no. 2, pp. 201-220 | DOI | MR | Zbl
[20] Variational Analysis and Applications, Springer Monographs in Mathematics, Springer, 2018, xix+622 pages | DOI | Zbl
[21] Stochastic Programming, Kluwer Academic Publishers, 1995 | DOI | Zbl
[22] Probabilistic programming, Stochastic Programming (A. Ruszczyński; A. Shapiro, eds.) (Handbooks in Operations Research and Management Science), Volume 10, Elsevier, 2003, pp. 267-351 | DOI | MR
[23] Variational Analysis, Grundlehren der Mathematischen Wissenschaften, 317, Springer, 2009, 734 pages | DOI | Zbl
[24] Implementable algorithm for stochastic optimization using sample average approximations, J. Optim. Theory Appl., Volume 122 (2004) no. 1, pp. 157-184 | DOI | MR | Zbl
[25] Extensions of stochastic optimization results to problems with system failure probability functions, J. Optim. Theory Appl., Volume 133 (2007) no. 1, pp. 1-18 | DOI | MR | Zbl
[26] Real and complex analysis, McGraw-Hill Series in Higher Mathematics, McGraw-Hill, 1987, xiv+416 pages | Zbl
[27] Derivatives of probability and Integral functions: General Theory and Examples, Encyclopedia of Optimization, Springer, 2009, pp. 658-663 | DOI
[28] A discussion of probability functions and constraints from a variational perspective, Set-Valued Var. Anal., Volume 28 (2020) no. 4, pp. 585-609 | DOI | MR | Zbl
[29] (Sub-)Differentiability of probability functions with elliptical distributions, Set-Valued Var. Anal., Volume 26 (2018) no. 4, pp. 887-910 | DOI | MR | Zbl
[30] Large-scale Unit Commitment under uncertainty: an updated literature survey, Ann. Oper. Res., Volume 271 (2018) no. 1, pp. 11-85 | DOI | MR | Zbl
[31] Gradient formulae for nonlinear probabilistic constraints with Gaussian and Gaussian-like distributions, SIAM J. Optim., Volume 24 (2014) no. 4, pp. 1864-1889 | DOI | MR | Zbl
[32] (Sub-)gradient formulae for probability functions of random inequality systems under Gaussian distribution, SIAM/ASA J. Uncertain. Quantif., Volume 5 (2017) no. 1, pp. 63-87 | DOI | MR | Zbl
[33] Generalized gradients for probabilistic/robust (probust) constraints, Optimization, Volume 69 (2020) no. 7-8, pp. 1451-1479 | DOI | MR | Zbl
[34] Derivatives of probability functions acting on parameter dependent unions of polyhedra, Set-Valued Var. Anal. (2021), pp. 1-33 | DOI
[35] Eventual convexity of probability constraints with elliptical distributions, Math. Program., Volume 175 (2019) no. 1-2, pp. 1-627 | DOI | MR | Zbl
[36] Generalized differentiation of probability functions acting on an infinite system of constraints, SIAM J. Optim., Volume 29 (2019) no. 3, pp. 2179-2210 | DOI | MR | Zbl
[37] Gradient formulae for nonlinear probabilistic constraints with non-convex quadratic forms, J. Optim. Theory Appl., Volume 185 (2020) no. 1, pp. 239-269 | DOI | MR | Zbl
[38] Generalized differentiation of probability functions: parameter dependent sets given by intersections of convex sets and complements of convex sets. (2021) (working paper)
Cited by Sources: