We consider structured minimization problems subject to smooth inequality constraints and present a flexible algorithm that combines interior point (IP) and proximal gradient schemes. While traditional IP methods cannot cope with nonsmooth objective functions and proximal algorithms cannot handle complicated constraints, their combined usage is shown to successfully compensate the respective shortcomings. We provide a theoretical characterization of the algorithm and its asymptotic properties, deriving convergence results for fully nonconvex problems, thus bridging the gap with previous works that successfully addressed the convex case. Our interior proximal gradient algorithm benefits from warm starting, generates strictly feasible iterates with decreasing objective value, and returns after finitely many iterations a primal-dual pair approximately satisfying suitable optimality conditions. As a byproduct of our analysis of proximal gradient iterations we demonstrate that a slight refinement of traditional backtracking techniques waives the need for upper bounding the stepsize sequence, as required in existing results for the nonconvex setting.
Revised:
Accepted:
Published online:
DOI: 10.5802/ojmo.30
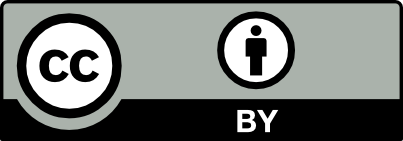
@article{OJMO_2024__5__A3_0, author = {Alberto De Marchi and Andreas Themelis}, title = {An interior proximal gradient method for nonconvex optimization}, journal = {Open Journal of Mathematical Optimization}, eid = {3}, pages = {1--22}, publisher = {Universit\'e de Montpellier}, volume = {5}, year = {2024}, doi = {10.5802/ojmo.30}, mrnumber = {4770119}, zbl = {07893308}, language = {en}, url = {https://ojmo.centre-mersenne.org/articles/10.5802/ojmo.30/} }
TY - JOUR AU - Alberto De Marchi AU - Andreas Themelis TI - An interior proximal gradient method for nonconvex optimization JO - Open Journal of Mathematical Optimization PY - 2024 SP - 1 EP - 22 VL - 5 PB - Université de Montpellier UR - https://ojmo.centre-mersenne.org/articles/10.5802/ojmo.30/ DO - 10.5802/ojmo.30 LA - en ID - OJMO_2024__5__A3_0 ER -
%0 Journal Article %A Alberto De Marchi %A Andreas Themelis %T An interior proximal gradient method for nonconvex optimization %J Open Journal of Mathematical Optimization %D 2024 %P 1-22 %V 5 %I Université de Montpellier %U https://ojmo.centre-mersenne.org/articles/10.5802/ojmo.30/ %R 10.5802/ojmo.30 %G en %F OJMO_2024__5__A3_0
Alberto De Marchi; Andreas Themelis. An interior proximal gradient method for nonconvex optimization. Open Journal of Mathematical Optimization, Volume 5 (2024), article no. 3, 22 p. doi : 10.5802/ojmo.30. https://ojmo.centre-mersenne.org/articles/10.5802/ojmo.30/
[1] A Bregman Forward-Backward Linesearch Algorithm for Nonconvex Composite Optimization: Superlinear Convergence to Nonisolated Local Minima, SIAM J. Optim., Volume 31 (2021) no. 1, pp. 653-685 | DOI | MR | Zbl
[2] Regularized symmetric indefinite systems in interior point methods for linear and quadratic optimization, Optim. Methods Softw., Volume 11 (1999) no. 1–4, pp. 275-302 | DOI | MR | Zbl
[3] A Mixed Logarithmic Barrier-Augmented Lagrangian Method for Nonlinear Optimization, J. Optim. Theory Appl., Volume 173 (2017) no. 2, pp. 523-547 | DOI | MR | Zbl
[4] FOM – a MATLAB toolbox of first-order methods for solving convex optimization problems, Optim. Methods Softw., Volume 34 (2019) no. 1, pp. 172-193 | DOI | MR | Zbl
[5] SPIRAL: A Superlinearly Convergent Incremental Proximal Algorithm for Nonconvex Finite Sum Minimization, Comput. Optim. Appl., Volume 88 (2024) no. 1, pp. 71-106 | DOI | MR | Zbl
[6] Nonlinear Programming, Athena Scientific, 1999 | MR
[7] Practical Augmented Lagrangian Methods for Constrained Optimization, Society for Industrial and Applied Mathematics, 2014 | DOI | MR
[8] First order methods beyond convexity and Lipschitz gradient continuity with applications to quadratic inverse problems, SIAM J. Optim., Volume 28 (2018) no. 3, pp. 2131-2151 | DOI | MR | Zbl
[9] An interior point method for nonlinear constrained derivative-free optimization (2022) | arXiv
[10] Computing the proximity operator of the norm with , IET Signal Process., Volume 10 (2016) no. 5, pp. 557-565 | DOI
[11] A Proximal Interior Point Algorithm with Applications to Image Processing, J. Math. Imaging Vis., Volume 62 (2020) no. 6, pp. 919-940 | DOI | MR | Zbl
[12] A penalty-interior-point algorithm for nonlinear constrained optimization, Math. Program. Comput., Volume 4 (2012) no. 2, pp. 181-209 | DOI | MR | Zbl
[13] Proximal gradient methods beyond monotony, Journal of Nonsmooth Analysis and Optimization, Volume 4 (2023) | DOI | MR
[14] Constrained composite optimization and augmented Lagrangian methods, Math. Program., Volume 201 (2023) no. 1, pp. 863-896 | DOI | MR | Zbl
[15] Proximal Gradient Algorithms under Local Lipschitz Gradient Continuity: A Convergence and Robustness Analysis of PANOC, J. Optim. Theory Appl., Volume 194 (2022) no. 3, pp. 771-794 | DOI | MR | Zbl
[16] Nonlinear Programming: Sequential Unconstrained Minimization Techniques, John Wiley & Sons, 1968 | MR
[17] Interior Methods for Nonlinear Optimization, SIAM Rev., Volume 44 (2002) no. 4, pp. 525-597 | DOI | MR | Zbl
[18] The logarithmic potential method of convex programming (1955) (Technical report)
[19] On projected Newton barrier methods for linear programming and an equivalence to Karmarkar’s projective method, Math. Program., Volume 36 (1986) no. 2, pp. 183-209 | DOI | MR | Zbl
[20] Interior point methods 25 years later, Eur. J. Oper. Res., Volume 218 (2012) no. 3, pp. 587-601 | DOI | MR | Zbl
[21] Convergence Properties of Monotone and Nonmonotone Proximal Gradient Methods Revisited, J. Optim. Theory Appl., Volume 195 (2022) no. 2, pp. 624-646 | DOI | MR | Zbl
[22] A new polynomial-time algorithm for linear programming, Combinatorica, Volume 4 (1984) no. 4, pp. 373-395 | DOI | MR
[23] A polynomial algorithm in linear programming, Sov. Math., Dokl., Volume 20 (1979), pp. 191-194
[24] Riemannian Interior Point Methods for Constrained Optimization on Manifolds, J. Optim. Theory Appl., Volume 201 (2024) no. 1, pp. 433-469 | DOI | MR | Zbl
[25] Bregman Finito/MISO for nonconvex regularized finite sum minimization without Lipschitz gradient continuity, SIAM J. Optim., Volume 32 (2022) no. 3, pp. 2230-2262 | DOI | MR | Zbl
[26] On the convergence of adaptive first order methods: proximal gradient and alternating minimization algorithms (2023) | arXiv
[27] Adaptive proximal algorithms for convex optimization under local Lipschitz continuity of the gradient (2023) | arXiv
[28] An ADMM-based interior-point method for large-scale linear programming, Optim. Methods Softw., Volume 36 (2021) no. 2–3, pp. 389-424 | DOI | MR | Zbl
[29] Simple Algorithms for Optimization on Riemannian Manifolds with Constraints, Appl. Math. Optim., Volume 82 (2020) no. 3, pp. 949-981 | DOI | MR | Zbl
[30] Relatively Smooth Convex Optimization by First-Order Methods, and Applications, SIAM J. Optim., Volume 28 (2018) no. 1, pp. 333-354 | DOI | MR | Zbl
[31] Solving Mixed-Integer Nonlinear Programs by QP-Diving (2012) (Preprint ANL/MCS-P2071-0312) (Technical report)
[32] Adaptive Gradient Descent without Descent, Proceedings of the 37th International Conference on Machine Learning, Volume 119, PMLR (2020), pp. 6702-6712
[33] Adaptive Proximal Gradient Method for Convex Optimization (2023) | arXiv
[34] Non-Negative Principal Component Analysis: Message Passing Algorithms and Sharp Asymptotics, IEEE Trans. Inf. Theory, Volume 62 (2016) no. 3, pp. 1458-1484 | DOI | MR | Zbl
[35] Variational Analysis and Applications, Springer, 2018 | DOI | MR
[36] Benchmarking Derivative-Free Optimization Algorithms, SIAM J. Optim., Volume 20 (2009) no. 1, pp. 172-191 | DOI | MR | Zbl
[37] Interior-Point Polynomial Algorithms in Convex Programming, Society for Industrial and Applied Mathematics, 1994 | DOI | MR
[38] Variational analysis, Grundlehren der Mathematischen Wissenschaften, 317, Springer, 1998 | DOI
[39] The Variable Metric Forward-Backward Splitting Algorithm Under Mild Differentiability Assumptions, SIAM J. Optim., Volume 27 (2017) no. 4, pp. 2153-2181 | DOI | MR | Zbl
[40] OpEn: Code Generation for Embedded Nonconvex Optimization, IFAC-PapersOnLine, Volume 53 (2020) no. 2, pp. 6548-6554 (21st IFAC World Congress) | DOI
[41] Forward-Backward Envelope for the Sum of Two Nonconvex Functions: Further Properties and Nonmonotone Linesearch Algorithms, SIAM J. Optim., Volume 28 (2018) no. 3, pp. 2274-2303 | DOI | MR | Zbl
[42] Interior-proximal primal-dual methods, Appl. Anal. Optim., Volume 3 (2019) no. 1, pp. 1-28 | MR | Zbl
[43] An Interior-Point Algorithm for Nonconvex Nonlinear Programming, Comput. Optim. Appl., Volume 13 (1999) no. 1, pp. 231-252 | DOI | MR | Zbl
[44] On the implementation of an interior-point filter line-search algorithm for large-scale nonlinear programming, Math. Program., Volume 106 (2006) no. 1, pp. 25-57 | DOI | MR | Zbl
[45] A Bregman inertial forward-reflected-backward method for nonconvex minimization, J. Glob. Optim., Volume 89 (2023) no. 2, pp. 327-354 | DOI | MR | Zbl
[46] The interior-point revolution in optimization: history, recent developments, and lasting consequences, Bull. Am. Math. Soc., Volume 42 (2005) no. 1, pp. 39-56 | DOI | MR | Zbl
[47] Primal-Dual Interior-Point Methods, Society for Industrial and Applied Mathematics, 1997 | DOI | MR
[48] Regularization: A Thresholding Representation Theory and a Fast Solver, IEEE Trans. Neural Netw. Learn. Syst., Volume 23 (2012) no. 7, pp. 1013-1027 | DOI
[49] Solving Constrained Variational Inequalities via a First-order Interior Point-based Method, The Eleventh International Conference on Learning Representations (ICLR) (2023)
Cited by Sources: