Consider the problem of minimizing functions that are Lipschitz and convex, but not necessarily differentiable. We construct a function from this class for which the iterate of subgradient descent has error . This matches a known upper bound of . We prove analogous results for functions that are additionally strongly convex. There exists such a function for which the error of the iterate of subgradient descent has error , matching a known upper bound of . These results resolve a question posed by Shamir (2012).
Revised:
Accepted:
Published online:
DOI: 10.5802/ojmo.31
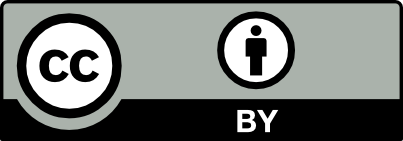
@article{OJMO_2024__5__A4_0, author = {Nicholas J. A. Harvey and Chris Liaw and Sikander Randhawa}, title = {Tight analyses for subgradient descent {I:} {Lower} bounds}, journal = {Open Journal of Mathematical Optimization}, eid = {4}, pages = {1--17}, publisher = {Universit\'e de Montpellier}, volume = {5}, year = {2024}, doi = {10.5802/ojmo.31}, mrnumber = {4779171}, zbl = {07939164}, language = {en}, url = {https://ojmo.centre-mersenne.org/articles/10.5802/ojmo.31/} }
TY - JOUR AU - Nicholas J. A. Harvey AU - Chris Liaw AU - Sikander Randhawa TI - Tight analyses for subgradient descent I: Lower bounds JO - Open Journal of Mathematical Optimization PY - 2024 SP - 1 EP - 17 VL - 5 PB - Université de Montpellier UR - https://ojmo.centre-mersenne.org/articles/10.5802/ojmo.31/ DO - 10.5802/ojmo.31 LA - en ID - OJMO_2024__5__A4_0 ER -
%0 Journal Article %A Nicholas J. A. Harvey %A Chris Liaw %A Sikander Randhawa %T Tight analyses for subgradient descent I: Lower bounds %J Open Journal of Mathematical Optimization %D 2024 %P 1-17 %V 5 %I Université de Montpellier %U https://ojmo.centre-mersenne.org/articles/10.5802/ojmo.31/ %R 10.5802/ojmo.31 %G en %F OJMO_2024__5__A4_0
Nicholas J. A. Harvey; Chris Liaw; Sikander Randhawa. Tight analyses for subgradient descent I: Lower bounds. Open Journal of Mathematical Optimization, Volume 5 (2024), article no. 4, 17 p. doi : 10.5802/ojmo.31. https://ojmo.centre-mersenne.org/articles/10.5802/ojmo.31/
[1] Convexity and optimization in Banach spaces, Springer, 2012 | DOI | MR
[2] Projection Algorithms and Monotone Operators, Ph. D. Thesis, Simon Fraser University (1996)
[3] Convex Analysis and Monotone Operator Theory in Hilbert Spaces, Springer, 2017 | DOI | MR
[4] Introduction to Online Convex Optimization, Found. Trends Optim., Volume 2 (2015) no. 3–4
[5] Logarithmic regret algorithms for online convex optimization, Mach. Learn., Volume 69 (2007) no. 2-3, pp. 169-192 | DOI | Zbl
[6] Beyond the regret minimization barrier: optimal algorithms for stochastic strongly-convex optimization, J. Mach. Learn. Theory, Volume 15 (2014) no. 1, pp. 2489-2512 | MR | Zbl
[7] Convex Analysis and Minimization Algorithms I, Springer, 1996
[8] Making the Last Iterate of SGD Information Theoretically Optimal, Conference on Learning Theory (2019), pp. 1752-1755
[9] A simpler approach to obtaining an convergence rate for the projected stochastic subgradient method (2012) | arXiv
[10] Problem complexity and method efficiency in optimization, John Wiley & Sons, 1983 | MR
[11] Quasi-monotone Subgradient Methods for Nonsmooth Convex Minimization, J. Optim. Theory Appl., Volume 165 (2015) no. 3, pp. 917-940 | DOI | MR | Zbl
[12] Acceleration of stochastic approximation by averaging, SIAM J. Control Optim., Volume 30 (1992) no. 4, pp. 838-855 | DOI | MR | Zbl
[13] Making gradient descent optimal for strongly convex stochastic optimization, Proceedings of ICML (2012)
[14] Convex Analysis, Princeton University Press, 1970 | DOI | MR
[15] Efficient estimations from a slowly convergent Robbins-Monro process (1988) (Technical report)
[16] Open Problem: Is Averaging Needed for Strongly Convex Stochastic Gradient Descent?, Proceedings of the 25th Annual Conference on Learning Theory (Proceedings of Machine Learning Research), Volume 23, 2012, p. 47.1-47.3
[17] Stochastic Gradient Descent for Non-smooth Optimization: Convergence Results and Optimal Averaging Schemes, Proceedings of the 30th International Conference on Machine Learning (Proceedings of Machine Learning Research), Volume 28, 2013, pp. 71-79
Cited by Sources: