A memory-efficient solution framework is proposed for the cardinality-constrained structured data-fitting problem. Dual-based atom-identification rules reveal the structure of the optimal primal solution from near-optimal dual solutions, which allows for a simple and computationally efficient algorithm that translates any feasible dual solution into a primal solution satisfying the cardinality constraint. Rigorous guarantees bound the quality of a near-optimal primal solution given any dual-based method that generates dual iterates converging to an optimal dual solution. Numerical experiments on real-world datasets support the analysis and demonstrate the efficiency of the proposed approach.
Revised:
Accepted:
Published online:
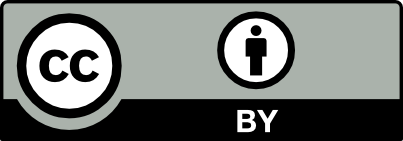
@article{OJMO_2024__5__A2_0, author = {Zhenan Fan and Huang Fang and Michael P. Friedlander}, title = {Cardinality-constrained structured data-fitting problems}, journal = {Open Journal of Mathematical Optimization}, eid = {2}, pages = {1--21}, publisher = {Universit\'e de Montpellier}, volume = {5}, year = {2024}, doi = {10.5802/ojmo.27}, language = {en}, url = {https://ojmo.centre-mersenne.org/articles/10.5802/ojmo.27/} }
TY - JOUR AU - Zhenan Fan AU - Huang Fang AU - Michael P. Friedlander TI - Cardinality-constrained structured data-fitting problems JO - Open Journal of Mathematical Optimization PY - 2024 SP - 1 EP - 21 VL - 5 PB - Université de Montpellier UR - https://ojmo.centre-mersenne.org/articles/10.5802/ojmo.27/ DO - 10.5802/ojmo.27 LA - en ID - OJMO_2024__5__A2_0 ER -
%0 Journal Article %A Zhenan Fan %A Huang Fang %A Michael P. Friedlander %T Cardinality-constrained structured data-fitting problems %J Open Journal of Mathematical Optimization %D 2024 %P 1-21 %V 5 %I Université de Montpellier %U https://ojmo.centre-mersenne.org/articles/10.5802/ojmo.27/ %R 10.5802/ojmo.27 %G en %F OJMO_2024__5__A2_0
Zhenan Fan; Huang Fang; Michael P. Friedlander. Cardinality-constrained structured data-fitting problems. Open Journal of Mathematical Optimization, Volume 5 (2024), article no. 2, 21 p. doi : 10.5802/ojmo.27. https://ojmo.centre-mersenne.org/articles/10.5802/ojmo.27/
[1] Linear convergence of a frank-wolfe type algorithm over trace-norm balls, NIPS’17: Proceedings of the 31st International Conference on Neural Information Processing Systems, Curran Associates Inc., 2017, pp. 6191-6200
[2] ADMM for Regularized Optimization Problems and Applications Oriented Input Design for MPC, 2012 (licentiate thesis, Stockholm, Sweden)
[3] Foundations of gauge and perspective duality, SIAM J. Optim., Volume 28 (2018) no. 3, pp. 2406-2434 | DOI | MR | Zbl
[4] Level-set methods for convex optimization, Math. Program., Volume 174 (2018) no. 1-2, pp. 359-390 | DOI | MR | Zbl
[5] Convex multi-task feature learning, Mach. Learn., Volume 73 (2008) no. 3, pp. 243-272 | DOI | Zbl
[6] Safe screening rules for -regression, Proceedings of International Conference on Machine Learning, 2020 (https://arxiv.org/abs/2004.08773)
[7] Fast oscar and owl with safe screening rules, Proceedings of International Conference on Machine Learning, 2020 (https://arxiv.org/abs/2006.16433)
[8] A fast iterative shrinkage-thresholding algorithm for linear inverse problems, SIAM J. Imaging Sci., Volume 2 (2009) no. 1, pp. 183-202 | DOI | MR | Zbl
[9] Probing the Pareto frontier for basis pursuit solutions, SIAM J. Sci. Comput., Volume 31 (2008) no. 2, pp. 890-912 | DOI | MR | Zbl
[10] Sparse optimization with least-squares constraints, SIAM J. Optim., Volume 21 (2011) no. 4, pp. 1201-1229 | DOI | MR | Zbl
[11] Algorithm 890: Sparco: A testing framework for sparse reconstruction, ACM Trans. Math. Softw., Volume 35 (2008) no. 4, 29, 16 pages | DOI
[12] Julia: A fresh approach to numerical computing, SIAM Rev., Volume 59 (2017) no. 1, pp. 65-98 | DOI | MR | Zbl
[13] Dynamic screening: Accelerating first-order algorithms for the lasso and group-lasso, IEEE Trans. Signal Process., Volume 63 (2015) no. 19, pp. 5121-5132 | DOI | MR | Zbl
[14] On the identification of active constraints, SIAM J. Numer. Anal., Volume 25 (1988) no. 5, pp. 1197-1211 | DOI | MR | Zbl
[15] Phase retrieval via matrix completion, SIAM J. Imaging Sci., Volume 6 (2013) no. 1, pp. 199-225 | DOI | MR | Zbl
[16] Robust principal component analysis?, J. Assoc. Comput. Mach., Volume 58 (2011) no. 3, 11, 37 pages | MR | Zbl
[17] Matrix Completion With Noise, Proc. IEEE, Volume 98 (2010) no. 6, pp. 925-936 | DOI
[18] Exact matrix completion via convex optimization, Commun. ACM, Volume 55 (2012) no. 6, pp. 111-119 | DOI
[19] Robust uncertainty principles: exact signal reconstruction from highly incomplete frequency information, IEEE Trans. Inf. Theory, Volume 52 (2006) no. 2, pp. 489-509 | DOI | MR | Zbl
[20] Phaselift: Exact and stable signal recovery from magnitude measurements via convex programming, Commun. Pure Appl. Math., Volume 66 (2013) no. 8, pp. 1241-1274 | DOI | MR | Zbl
[21] The convex geometry of linear inverse problems, Found. Comput. Math., Volume 12 (2012) no. 6, pp. 805-849 | DOI | MR | Zbl
[22] Atomic decomposition by basis pursuit, SIAM J. Sci. Comput., Volume 20 (1998) no. 1, pp. 33-61 | DOI | MR
[23] An optimal-storage approach to semidefinite programming using approximate complementarity, SIAM J. Optim., Volume 31 (2021) no. 4, pp. 2695-2725 | DOI | MR | Zbl
[24] Compressed sensing, IEEE Trans. Inf. Theory, Volume 52 (2006) no. 4, pp. 1289-1306 | DOI | MR | Zbl
[25] For most large underdetermined systems of linear equations the minimal -norm near-solution approximates the sparsest near-solution, 2006 (technical report, Department of Statistics, Stanford University, Stanford)
[26] Safe feature elimination in sparse supervised learning, Pac. J. Optim., Volume 8 (2012) no. 4, pp. 667-698 | MR | Zbl
[27] Polar Deconvolution of Mixed Signals, IEEE Trans. Signal Process., Volume 70 (2022), pp. 2713-2727 | MR
[28] Atomic decomposition via polar alignment: The geometry of structured optimization, Found. Trends Optim., Volume 3 (2020) no. 4, pp. 280-366
[29] Bundle methods for dual atomic pursuit, Asilomar Conference on Signals, Systems, and Computers (ACSSC 2019), IEEE, 2019, pp. 264-270
[30] Approximations for partially coherent optical imaging systems, 1998 (technical report)
[31] An algorithm for quadratic programming, Nav. Res. Logist., Volume 3 (1956) no. 1-2, pp. 95-110 | DOI | MR
[32] Low-rank spectral optimization via gauge duality, SIAM J. Sci. Comput., Volume 38 (2016) no. 3, p. A1616-A1638 | DOI | MR | Zbl
[33] Exact regularization of convex programs, SIAM J. Optim., Volume 18 (2007) no. 4, pp. 1326-1350 | DOI | MR | Zbl
[34] From few to many: Illumination cone models for face recognition under variable lighting and pose, IEEE Trans. Pattern Anal. Mach. Intell., Volume 23 (2001) no. 6, pp. 643-660 | DOI
[35] Identifying active manifolds in regularization problems, Fixed-Point Algorithms for Inverse Problems in Science and Engineering (Springer Optimization and Its Applications), Volume 49, Springer, 2011, pp. 261-271 | DOI | MR | Zbl
[36] Identifying active constraints via partial smoothness and prox-regularity, J. Convex Anal., Volume 11 (2004) no. 2, pp. 251-266 | MR | Zbl
[37] A spectral bundle method for semidefinite programming, SIAM J. Optim., Volume 10 (2000) no. 3, pp. 673-696 | DOI | MR | Zbl
[38] Fundamentals of Convex Analysis, Grundlehren. Text Editions, Springer, 2001 | DOI | Zbl
[39] Scaling up sparse support vector machines by simultaneous feature and sample reduction, ICML’17: Proceedings of the 34th International Conference on Machine Learning, JMLR, 2017, pp. 4016-4025
[40] Nuclear norm minimization via active subspace selection, ICML’14: Proceedings of the 31st International Conference on International Conference on Machine Learning, JMLR, 2014, pp. 575-583
[41] Revisiting Frank–Wolfe: Projection-free sparse convex optimization, ICML’13: Proceedings of the 30th International Conference on International Conference on Machine Learning, JMLR, 2013, pp. 427-435
[42] On the duality of strong convexity and strong smoothness: Learning applications and matrix regularization (2009) (unpublished manuscript, https://home.ttic.edu/~shai/papers/KakadeShalevTewari09.pdf)
[43] A method for large-scale -regularized logistic regression, AAAI’07: Proceedings of the 22nd national conference on Artificial intelligence, AAAI Press, 2007
[44] Explicit sensor network localization using semidefinite representations and facial reductions, SIAM J. Optim., Volume 20 (2010) no. 5, pp. 2679-2708 | DOI | MR | Zbl
[45] A screening rule for -regularized Ising model estimation, Adv. Neural Inf. Process. Syst., Volume 30 (2017), pp. 720-731 (NIPS 2017)
[46] Linearized alternating direction method with adaptive penalty for low rank representation, NIPS’11: Proceedings of the 24th International Conference on Neural Information Processing Systems, Curran Associates Inc., 2011, pp. 612-620
[47] Safe screening with variational inequalities and its application to lasso, ICML’14: Proceedings of the 31st International Conference on International Conference on Machine Learning, JMLR, 2014, pp. 289-297
[48] Spectral regularization algorithms for learning large incomplete matrices, J. Mach. Learn. Res., Volume 11 (2010), pp. 2287-2322 | MR | Zbl
[49] High dimensional graphs and variable selection with the lasso, Ann. Stat., Volume 34 (2006) no. 3, pp. 1436-1462 | DOI | MR | Zbl
[50] Gap safe screening rules for sparse-group lasso, NIPS’16: Proceedings of the 30th International Conference on Neural Information Processing Systems, Curran Associates Inc., 2016, pp. 388-396
[51] Gap safe screening rules for sparsity enforcing penalties, J. Mach. Learn. Res., Volume 18 (2017), 128, 33 pages | MR | Zbl
[52] “active-set complexity” of proximal gradient: How long does it take to find the sparsity pattern?, Optim. Lett., Volume 13 (2019) no. 4, pp. 645-655 | DOI | MR | Zbl
[53] A second-order bundle method to minimize the maximum eigenvalue function, Math. Program., Volume 89 (2000) no. 1, pp. 1-33 | DOI | MR | Zbl
[54] Screening rules for convex problems (2015) | arXiv
[55] Fast maximum margin matrix factorization for collaborative prediction, ICML’05: Proceedings of the 22nd international conference on Machine learning, ACM Press, 2005, pp. 713-719 | DOI
[56] Convex Analysis, Princeton Mathematical Series, 28, Princeton University Press, 1970 | DOI
[57] Regression shrinkage and selection via the lasso, J. R. Stat. Soc., Ser. B, Stat. Methodol., Volume 58 (1996) no. 1, pp. 267-288 | MR | Zbl
[58] Convex recovery of a structured signal from independent random linear measurements, Sampling Theory, a Renaissance (Applied and Numerical Harmonic Analysis), Birkhäuser/Springer, 2015, pp. 67-101 | DOI | Zbl
[59] A safe screening rule for sparse logistic regression, NIPS’14: Proceedings of the 27th International Conference on Neural Information Processing Systems, MIT Press, 2014, pp. 1053-1061
[60] Lasso screening rules via dual polytope projection, NIPS’13: Proceedings of the 26th International Conference on Neural Information Processing Systems, Curran Associates Inc., 2013, pp. 1070-1078
[61] Screening tests for lasso problems, IEEE Trans. Pattern Anal. Mach. Intell., Volume 39 (2017) no. 5, pp. 1008-1027 | DOI
[62] Model selection and estimation in regression with grouped variables, J. R. Stat. Soc., Ser. B, Stat. Methodol., Volume 68 (2006) no. 1, pp. 49-67 | DOI | MR | Zbl
[63] Gradient hard thresholding pursuit, J. Mach. Learn. Res., Volume 18 (2018), 166, 43 pages | MR | Zbl
Cited by Sources: